Dear Fellow Scholars, this is Two Minute Papers with Károly Zsolnai-Fehér.
Today, we will listen to a new AI from DeepMind that is capable of creating beautiful piano
music.
Because there are many other algorithms that do that, to put things into perspective, let's
talk about the two key differentiating factors that set this method apart from previously
existing techniques.
One, music is typically learned from high-level representations, such as the score or MIDI
data.
This is a precise representation of what needs to be played, but they don't tell us how to
play them.
These small nuances are what makes the music come alive, and this is exactly what is missing
from most of the synthesis techniques.
This new method is able to learn these structures and generates not midi signals but raw audio
waveforms.
And two, it is better at retaining stylistic consistency.
Most previous techniques create music that is consistent on a shorter time-scale, but
do not take into consideration what was played 30 seconds ago, and therefore they lack the
high-level structure that is the hallmark of quality songwriting.
However, this new method shows stylistic consistency over longer time periods.
Let's give it a quick listen and talk about the architecture of this learning algorithm
after that.
While we listen, I'll show you the composers it has learned from to produce this.
I have never heard any AI-generated music before with such articulation and the harmonies
are also absolutely amazing.
Truly stunning results.
It uses an architecture that goes by the name autoregressive discrete autoencoder.
This contains an encoder module that takes a raw audio waveform and compresses it down
into an internal representation, where the decoder part is responsible for reconstructing
the raw audio from this internal representation.
Both of them are neural networks.
The autoregressive part means that the algorithm looks at previous time steps in the learned
audio signals when producing new notes, and is implemented in the encoder module.
Essentially, this is what gives the algorithm longer-term memory to remember what it played
earlier.
As you have seen the dataset the algorithm learned from as the music was playing, I am
also really curious how we can exert artistic control over the output by changing the dataset.
Essentially, you can likely change what the student learns by changing the textbooks used
to teach them.
For now, let's marvel at one more sound sample.
This is already incredible, and I can only imagine what we will be able to do not ten
years from now, just a year from now.
Thanks for watching and for your generous support, and I'll see you next time!
For more infomation >> DeepMind's AI Learns The Piano From The Masters of The Past - Duration: 4:00.-------------------------------------------
100 DIY Terrarium Ideas | DIY Garden - Duration: 10:26.
-------------------------------------------
TOP 10 BEST APPLICATIONS FOR ANDROID THAT YOU MAKE YOUR SMARTPHONE SMOOTHER - Duration: 5:24.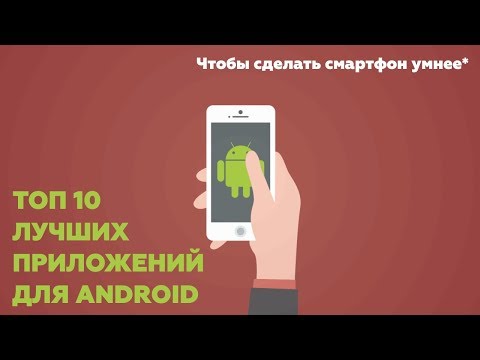
-------------------------------------------
Right Wing Pastor Warns: Stop Talking About Trump's Affairs Or Face God's Wrath! - Duration: 3:19.
Right wing evangelical pastor Franklin Graham, the son of the late Billy Graham has some
advice for those in the media who keep talking about Donald Trump and his affairs with porn
stars and Playboy playmates.
He says that if you continue to talk about these things and talk about the payoffs that
he made, then you are going to feel God's wrath.
That's right.
According to Franklin Graham, God is getting super pissed about all of us talking about
how horrible Donald Trump is, what a womanizer he is, the potentially illegal activity he
engaged in to cover all this up.
That makes God mad, so sayeth Franklin Graham.
Not the acts themselves, God's totally cool with that, probably would high five Donald
Trump if he got the chance, right Franklin?
But no, it's the people talking about it, trying to make it an issue.
We're the problems and we're the ones who should fear God's wrath.
Here's a couple of tweets that Mr. Franklin Graham sent out recently explaining this.
"Everyone in the media is talking about the just released tape and what the president
said or didn't say.
What he meant or didn't mean.
It is a good moment to point out that everyone should realize that every word that is spoken
or thought is recorded by God."
God's basically a much more powerful version of Michael Cohen, I guess.
He continues, "The Bible said it is appointed unto man once to die and then the judgment.
We won't be judged by media spin masters or forensic audio analysts, but you will be judged
by truth and righteousness by God himself.
Have you ever thought about what his verdict will be on your words?"
I would hope, personally, that God would say to me, "Hey, good job calling out those in
power who are screwing over those without power."
That's pretty much what the entire Bible was all about and thank you for actually doing
what the Bible said.
Now I may not be out there feeding the poor or helping the sick right now, but I am trying
to educate, as are most members of the media.
Some of them are in it for the profits, some of them are in it for the glory and the fame
and the narcissism that they have inside.
But most of us just want to expose the rampant corruption that's happening here in the United
States.
But I have a question for you, Mr. Graham.
If you have indeed read the Bible, you know that the one thing that pisses God off more
than anything is false prophets.
You know, and that's exactly what you are.
You're a charlatan.
You're a liar, you're a hypocrite because you were very, very interested in Bill Clinton's
sex life in the 1990s.
But now suddenly it's off limits when we're talking about even more illegal activity than
what Bill Clinton did?
These evangelicals have no ground to stand on anymore.
They have no credibility whatsoever and anyone in this country that believes a word that
Billy Graham, or Franklin Graham, excuse me, any one of these assholes out there has to
say, is absolutely following these false prophets straight to hell.
-------------------------------------------
Music & The Spoken Word - Live Stream July 29, 2018 - Duration: 56:52.
-------------------------------------------
Dance Moms: Dance Digest - "Brat Pack" (Season 3) | Lifetime - Duration: 4:52.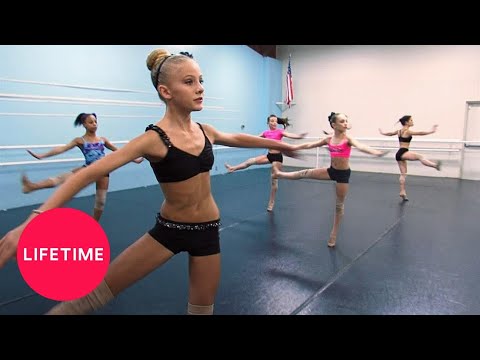
-------------------------------------------
Tony Stark Entry - Stark Tower Scene | Marvel's The Avengers (2012) - Duration: 4:58.
Good to go on this end. The rest is up to you.
You disconnected the transmission lines?
Are we off the grid?
Stark Tower is about to become a beacon
of self-sustaining clean energy.
Well, assuming the arc reactor takes over
and it actually works.
I assume.
Light her up.
How does it look?
Like Christmas, but with more me.
We've got to go wider on the public awareness campaign.
You need to do some press.
I'm in DC tomorrow,
I'm working on the zoning for the next three buildings.
Pepper, you're killing me. The moment, remember?
Enjoy the moment.
Get in here and I will.
Sir, Agent Coulson of S.H.I.E.L.D. Is on the line.
I'm not in.
I'm actually out.
Sir, I'm afraid he's insisting.
Grow a spine, Jarvis. I got a date.
Levels are holding steady, I think.
Of course they are. I was directly involved.
Which brings me to my next question.
How does it feel to be a genius?
Well, I really wouldn't know, now would I?
What do you mean? All this came from you.
No, all this came from that.
Give yourself some credit. Please.
Stark Tower is your baby.
Give yourself 12% of the credit.
12%?
An argument can be made for 15.
12%? My baby?
Well, I did do all the heavy lifting.
Literally, I lifted the heavy things.
And, sorry, but the security snafu?
That was on you.
- My private elevator... - You mean our elevator?
...it was teeming with sweaty workmen.
I'm going to pay for that comment about percentages
in some subtle way later, aren't I?
It's not going to be that subtle.
I'll tell you what.
The next building is gonna say "Potts" on the tower.
On the lease.
Call your mom. Can you bunk over?
Sir, the telephone.
I'm afraid my protocols are being overridden.
Mr Stark, we need to talk.
You have reached the life model decoy of Tony Stark.
- Please leave a message. - This is urgent.
Then leave it urgently.
Security breach. It's on you.
- Mr Stark. - Phil! Come in.
"Phil"?
I can't stay.
His first name is "Agent."
Come on in. We're celebrating.
Which is why he can't stay.
We need you to look this over as soon as possible.
I don't like being handed things.
That's fine, because I love to be handed things.
So, let's trade.
Thank you.
Official consulting hours are between 8 and 5
every other Thursday.
This isn't a consultation.
Is this about the Avengers?
Which I know nothing about.
The Avengers Initiative was scrapped, I thought.
And I didn't even qualify.
I didn't know that either.
Apparently I'm volatile, self-obsessed,
don't play well with others.
That I did know.
This isn't about personality profiles any more.
Whatever. Ms Potts, got a second?
Half a mo.
You know, I thought we were having a moment.
I was having 12% of a moment.
This seems serious. Phil's pretty shaken.
How would you know if it's... Why is he "Phil"?
What is all this?
This is
this.
I'm going to take the jet to DC tonight.
Tomorrow.
You have homework. You have a lot of homework.
Well, what if I didn't?
- Lf you didn't? - Yeah.
You mean when you've finished?
Well, then...
Square deal. Fly safe.
Work hard.
So, any chance you're driving by LaGuardia?
- I can drop you. - Fantastic.
I want to hear
about the cellist. Is that still a thing?
She moved back to Portland.
What? Boo!
-------------------------------------------
John Ronan's Charred Wood Courtyard House Frames Views Of Lake Michigan | Great Small House Design - Duration: 2:21.
John Ronan's Charred Wood Courtyard House Frames Views Of Lake Michigan
-------------------------------------------
Cal OES NEWS UPDATE: Statewide Fires Update July 29, 2018 - Duration: 1:42.
I'm Bryan May in the State Operations Center Cal OES headquarters, I just want
to give you an update on the largest of the fires that are burning across
California on this Sunday morning and there are about twelve large wildfires
burning it this morning. I'm gonna run down the top four of those
and we'll start at the northern part of the state and work our way down. So the
Carr Fire, that's the large one in Shasta County, over 89,000 acres
and still just five percent contained, latest number about five hundred
structures destroyed in that fire so far. Working our way down to the Mendocino
Complex fire this is a fire that spans both Mendocino and Lake counties, they
combined it into one, it was two fires originally, it's now one large fire
24,000 acres, 10% containment and overnight that fire caused a massive
power outage in those two counties Mendocino and Lake County at one point,
there were about 37,000 people without power as of this morning all but about
500 of those have been restored. In Mariposa County, the Ferguson Fire, that's
the one that's had blanketed the
Yosemite valley with smoke, the Ferguson
Fire over 53,000 acres now containment up to 30% and then in Riverside County,
the Cranston Fire, 13,130 acres, containment at 29%. All of these fires
still very dynamic with incredibly hot temperatures across the state so, firefighters
continue to work on people getting back to their homes as safely and as soon as
possible. You can continue to monitor all of our social media feeds Facebook
Twitter as well as our OES news.com We'll have updates throughout the day.
For all of us at Cal OES I'm Bryan May, thanks for watching.
-------------------------------------------
Karbala Kahini Bangla Episode 88||কারবালা কাহিনী পর্ব 88||SATV✔️ - Duration: 23:59.
Thank you so much for checking out this video.
Definitely subscribe for more videos
just like this hit that like button
if you've gotten value out of this video.
-------------------------------------------
How to Boost Metabolism - Secrets To Boost Metabolism Fast! - Duration: 3:29.
-------------------------------------------
[NEW] LUNAR ECLIPSE FIGHT |FIGHTING TIGER| - Duration: 3:10.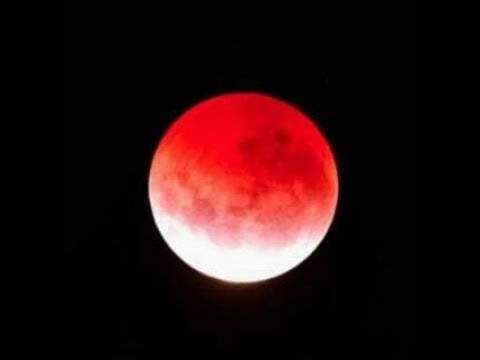
-------------------------------------------
চলছিল শ্যুটিং,হঠাৎ লন্ডভন্ড হয়ে গেল রানি রাসমণির সেট দেখুন ভিডিও|Rani Rashmoni Serial Shooting Set - Duration: 1:56.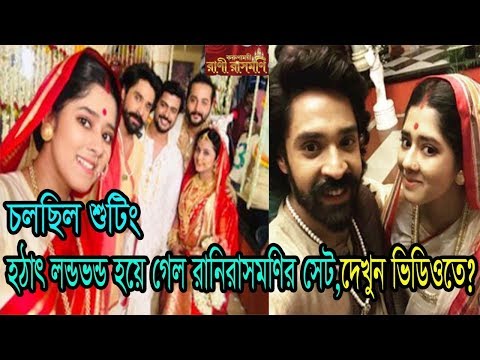
-------------------------------------------
[Vocaloid Studio] New studio!!! 歌愛ユキ(Kaai Yuki) - Popipo - Duration: 2:59.
Po-ppi-po-ppi-po-ppo-ppi-pou (x11)
Po-ppi-po-ppi-po-ppo-ppi
PI!!
Saa nome omae suki daro? Yasai juusu
Watashi ga kimeta ima kimeta
Dakara nonde watashi no yasai juusu
Kakaku wa nihyaku en
Soiya!! Soiya!!
Dossee!! Dossee!!
Soiya!! Soiya!!
Dossee!! Dossee!!
Maroyaka yasai juusu
Fuwa Fuwa Yasai Juusu
Ichiban osusume na no wa Midori no juusu
Po-ppi-po-ppi-po-ppo-ppi-pou (2x)
Bejitaburu na
Po-ppi-po-ppi-po-ppo-ppi-pou (2x)
Seimei afureta
Po-ppi-po-ppi-po-ppo-ppi-pou (2x)
Anata mo ima
Po-ppi-po-ppi-po-ppo-ppi-pou (2x)
Yasai juusu ga suki ni naru
PI!!
Let's take, You're lovin' it. "Vegetable juice."
You must love this drink, I've decided now
So take it now, with my true heart. "Vegetable juice."
It costs just 2 dollars
Come on! Come on!
Let's dance!
Come on! Come on!
Let's dance!
Mellow and mellow vegetable juice
Creamy and creamy vegetable juice
I guess you should like The best, this one
Big, bright red juice!
Po-ppi-po-ppi-po-ppo-ppi-pou (2x)
We are vegetaria-a-a-an
Po-ppi-po-ppi-po-ppo-ppi-pou (2x)
Every vegetaria-a-a-a-an
Po-ppi-po-ppi-po-ppo-ppi-pou (2x)
Happy vegetaria-a-a-a-an
Po-ppi-po-ppi-po-ppo-ppi-pou (2x)
Y. A. S. A. I love you, so and I love you!!
-------------------------------------------
КАК МАРМОК ДЕЛАЕТ ПЕРЕХОДЫ?! СОЗДАНИЕ ТЕЛЕВИЗОРА, АНИМАЦИИ И Т.Д - Duration: 13:37.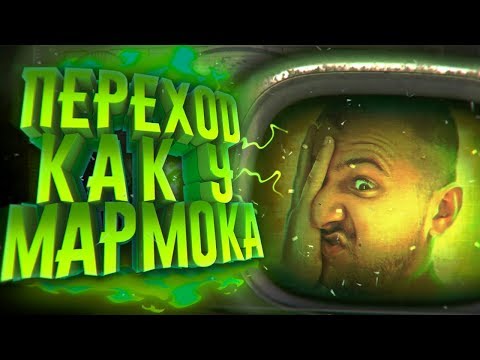
GUY WHO MAKES SUBTITLES, DIED BECAUSE OF LIGMA
-------------------------------------------
শাকিব খানের নামে মামলা করা উচিত !! bangla news - Duration: 3:45.
At the office of Motijheel office on Friday silence
Some buses and some rickshaws are in progress.
The movement of people is very less.
Passing the circular road of Motijheel, Tikatuli Road is seen to be a steep car.
Then at 6 pm, 15 minutes.
After 10 minutes, we saw the main reason for the traffic jam on this holiday.
After 10 minutes, we saw the main reason for the traffic jam on this holiday.
At the Motijheel Modhumita Cinema Hall broke the 3rd show.
More than a thousand viewers are watching movies.
Thousands of more visitors are waiting in front of the hall to see the 7th show.
Thousands of more visitors are waiting in front of the hall to see the 7th show.
The crowd of people in front of the movie hall.
The car can not move
Vanshul Abdur Rahman was excited about this hour for about an hour.
NTV said to the online, 'See what the situation?
You do not want to show on TV!
They will watch movies and we will be sitting in the jute this Friday.
The real culprit is Shakib Khan.
Should be sued in his name.
It is not such a situation when other heroes are playing the movies. "Others laughed at the traffic jam.
It is not such a situation when other heroes are playing the movies. "Others laughed at the traffic jam.
Going forward, there are thousands of people inside and outside the cinema hall.
The time is 6 to 30 minutes.
The time is 6 to 30 minutes.
The visitors were coming out from the cinema hall.
Many people are trying to enter the next show to see.
Cinema hall counter closed.
BlackBazaar (Black) is selling tickets.
Second seat of the hall is 300 taka, the first class ticket is sold at Tk 200.
Without making any allegations, the viewers of the movie are selling 150 taka for Taka 300 and Taka 100 for Taka 200.
Without making any allegations, the viewers of the movie are selling 150 taka for Taka 300 and Taka 100 for Taka 200.
Without making any allegations, the viewers of the movie are selling 150 taka for Taka 300 and Taka 100 for Taka 200.
It was not possible to pour out crowds to try to go in.
There are 1200 seats in the movie.
About three thousand viewers are in front of the hall.
About three thousand viewers are in front of the hall.
Although there is a Jonaki movie in Paltan, the same thing can be seen.
Counter off, thousands of visitors in front of the movie hall
Here, DC tickets for 90 taka are sold at Tk. 200.
And the ordinary class of Tk 70 is sold at Tk 150.
And the ordinary class of Tk 70 is sold at Tk 150.
In the meantime, the 7th show started.
Many people are returning home without getting tickets.
One such person came from Manu Mia family, a local grocery store owner, to see the pictures.
One such person came from Manu Mia family, a local grocery store owner, to see the pictures.
He said, 'The ticket counters are closed.
The sale of tickets to Blaay is at double the price.
Still did not get the ticket.
I do not usually watch movies.
But for the wife it is often come.
She has many fans of Shakib Khan.
Well, I did not get a ticket today.
After two days the viewers will lose a bit, then the price of the ticket will also decrease.
Yesterday, 109 films were released all over the country, 'Bhajan Alo Ray' directed by Jaydeep Mukherjee.
Yesterday, 109 films were released all over the country, 'Bhajan Alo Ray' directed by Jaydeep Mukherjee.
The film was produced by Kolkata-based producer SK Movies.
Shabanti and Pael Sarkar acted opposite Shakib Khan in the film.
Shabanti and Pael Sarkar acted opposite Shakib Khan in the film.
Không có nhận xét nào:
Đăng nhận xét